This course provides an overview of some different concepts underpinning Generative AI, their mathematical principles, and their applications in engineering. The focus will be on the practical implementation of generative AI including, neural networks, attention mechanism, and advanced deep learning models.

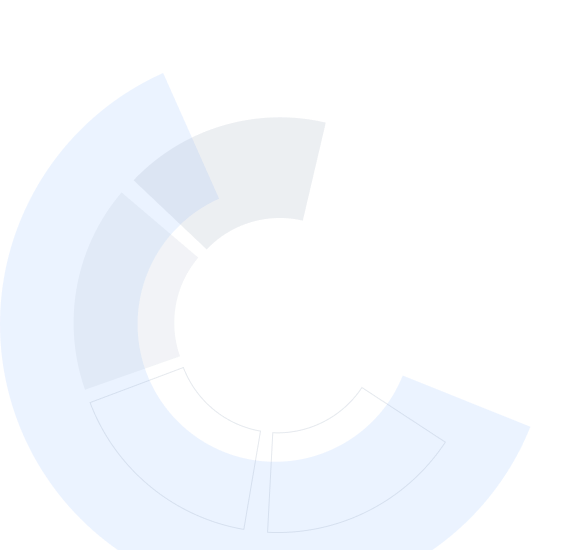
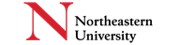
Generative AI: Foundations and Concepts

Instructor: Ramin Mohammadi
Access provided by New York State Department of Labor
Details to know

Add to your LinkedIn profile
9 assignments
April 2025
See how employees at top companies are mastering in-demand skills
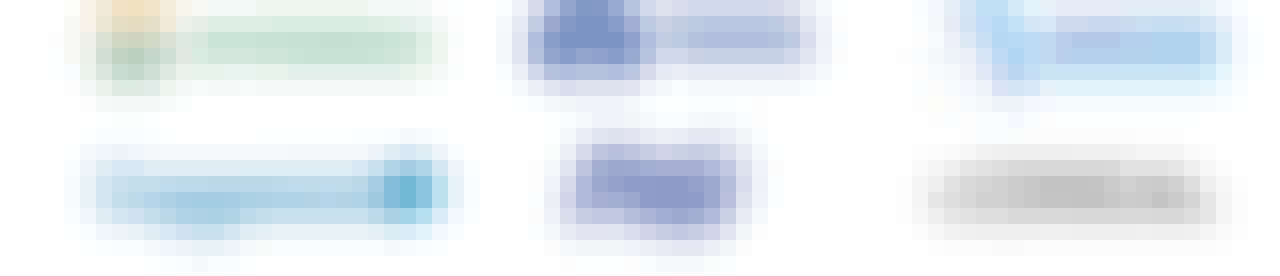
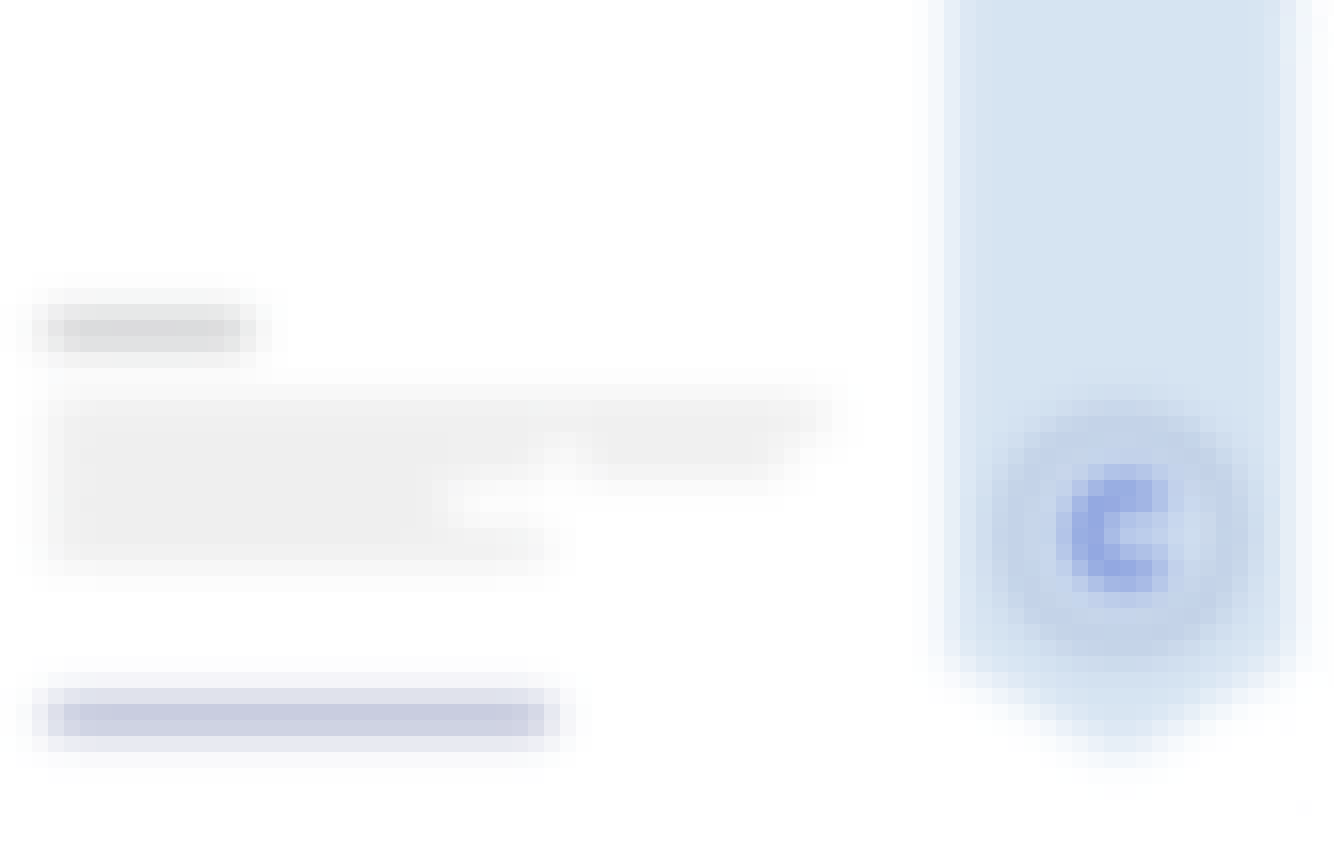
Earn a career certificate
Add this credential to your LinkedIn profile, resume, or CV
Share it on social media and in your performance review
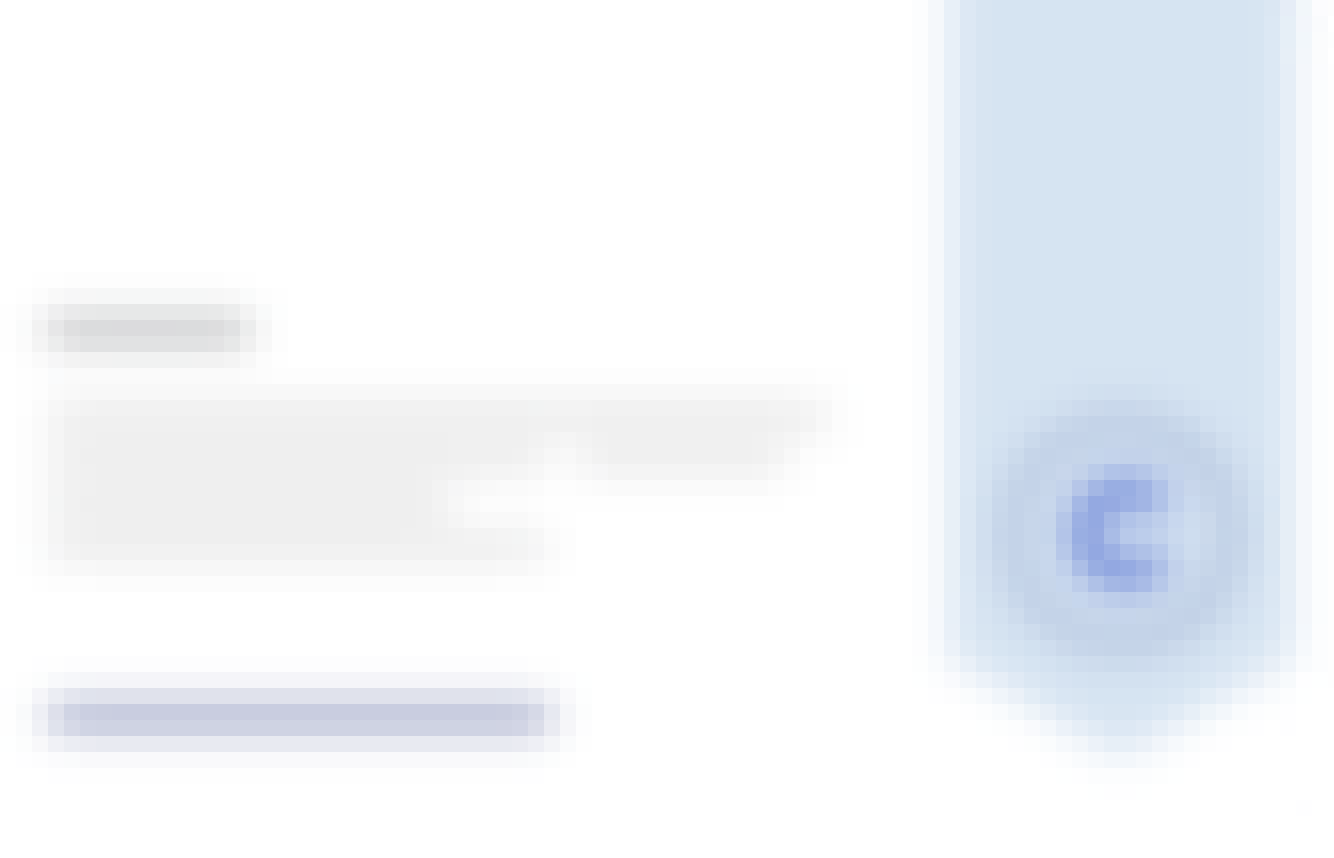
There are 4 modules in this course
In this module, you will explore the foundations of neural networks, including perceptrons, architectures, and learning algorithms. You will dive deeply into optimization methods critical for efficient training, focusing on advanced techniques like Newton’s and quasi-Newton methods, momentum, RMSProp, and Adam optimization algorithms.
What's included
6 videos15 readings2 assignments2 discussion prompts
This module guides you through the mathematical approaches to regularization techniques that enhance neural network generalization and prevent overfitting. You will analyze concepts including Stein’s unbiased risk estimator, eigen decomposition, ensemble methods, dropout mechanisms, and advanced normalization techniques such as batch normalization.
What's included
4 videos17 readings2 assignments1 discussion prompt
In this module, you will examine convolutional neural networks (CNNs), including convolution operations, parameter sharing, kernel methods, and multi-dimensional data structures. You'll explore advanced CNN architectures, regularization, normalization techniques, and the implications of random kernels on network learning behavior.
What's included
5 videos31 readings2 assignments1 discussion prompt
In this module, you will analyze the maths underpinning generative models and maximum likelihood estimation (MLE). You will explore divergence metrics such as Kullback-Leibler divergence, Bayesian network structures, and autoregressive modeling methods, focusing on their theoretical foundations and practical implications.
What's included
6 videos33 readings3 assignments1 discussion prompt
Instructor

Offered by
Why people choose Coursera for their career




Recommended if you're interested in Data Science
University of Colorado Boulder
Duke University
Google Cloud

Open new doors with Coursera Plus
Unlimited access to 10,000+ world-class courses, hands-on projects, and job-ready certificate programs - all included in your subscription
Advance your career with an online degree
Earn a degree from world-class universities - 100% online
Join over 3,400 global companies that choose Coursera for Business
Upskill your employees to excel in the digital economy